Structural Equation Modeling of Multiple Rater Data
Michael Eid, Christian Geiser, and Tobias Koch
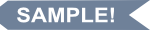
1.1 Advantages and Limitations of Self-Reports
1.2 Advantages and Limitations of Other Reports
1.21 Models of accuracy of other ratings
1.22 Sources of accuracy of other ratings
1.3 Usefulness of Multiple Rater Studies
1.31 Analyzing the validity of ratings
1.32 Improving the validity of inferences by multiple ratings
1.4 The Role of Measurement Models
1.5 Summary
1.6 Suggested Further Readings
2. Basic Methodological Concepts
2.1 Design Issues
2.1.1 Interchangeable and structurally different raters
2.1.2 Measurement designs
2.2 Confirmatory Factor Analysis of Multiple Rater Data
2.3 Stochastic Measurement Theory: Basic Ideas
2.3.1 Sampling process for structurally different raters
2.3.2 Sampling process for interchangeable raters
2.3.3 Differences between structurally different and interchangeable raters
2.4 Overview of the Present Book
2.5 Summary
2.6 Suggested Further Readings
3. Basic Models for Structurally Different Raters
3.1 Basic Decomposition of Observed Variables
3.2 Basic Model with Correlated First-Order Factors
3.2.1 Application of the MTMR model with correlated first-order factors: Loneliness and flourishing
3.3 Model with Indicator-Specific Factors
3.3.1 Application of the MTMR model with correlated first-order factors and indicator-specific factors: Loneliness and flourishing
3.3.2 Recommendations: Model Selection
3.4 Basic Model with Measurement Invariance Across Raters
3.4.1 Statistical tests for testing measurement invariance
3.4.2 Partial measurement invariance and measurement invariance in models with indicator-specific factors
3.4.3 How important is measurement invariance in multirater studies?
3.5 Application of the Models to Other Measurement Designs
3.6 Chapter Summary
3.7 Suggested Further Readings
4. Models with Method Factors for Structurally Different Raters
4.1 Basic CTC(M-1) Model
4.1.1 Choice of a reference rater group
4.1.2 Application of the CTC(M-1) model: Loneliness and flourishing
4.1.3 Comparing the CTC(M-1) model with the model with correlated first-order factors
4.2 Restricted CTC(M-1) Model as Reformulation of the Model with Correlated First-Order Factors
4.3 Restricted CTC(M-1) Model with Measurement Invariance Across Raters
4.4 CTC(M-1) Models with Indicator-Specific Effects
4.4.1 CTC(M-1) models with indicator-specific factors
4.4.2 CTC(M-1) model with indicator-specific traits
4.4.3. Choosing a CTC(M-1) model with indicator-specific effects
4.5 Alternative Models
4.5.1 Latent difference model
4.5.2 Latent means model
4.5.3 Reference rating as outcome model
4.6 Models with Covariates
4.7 Application of the Models to Other Measurement Designs
4.7.1 Analyzing round-robin data for structurally different raters
4.8 Chapter Summary
4.9 Suggested Further Readings
5. Single-Level CFA Models for Interchangeable Raters
5.1 The Interchangeable-Saturated (I-SAT) Model
5.2 Basic Decomposition
5.3 Basic Models with Correlated First-Order Factors
5.3.1 Adjustment of fit statistics with interchangeable models
5.3.2 Application of the basic model with correlated first-order factors with and without indicator-specific factors: Loneliness and flourishing
5.4 Models with Method Factors
5.4.1 Basic correlated traits-interchangeable methods (CTIM) model
5.4.2 Application of the basic CTIM model: Loneliness and flourishing
5.4.3 Restricted CTIM model
5.4.4 Application of the restricted CTIM model: Loneliness and flourishing
5.4.5 CTIM models with indicator-specific effects
5.5 Chapter Summary
5.6 Suggested Further Readings
6. Multilevel CFA Models for Interchangeable Raters
6.1 Multilevel Confirmatory Factor Analysis
6.1.1 Wide- versus long-format data
6.2 Basic Models with Correlated First-Order Factors and CTIM Models
6.2.1 Invariance constraints, variance decomposition, and coefficients
6.2.2 Application of CTIM models: Loneliness and flourishing
6.3 Practical Issues
6.4 Models for Round-Robin Designs with Interchangeable Raters
6.4.1 Analyzing round-robin data with interchangeable raters
6.4.2 Application to flourishing and loneliness data
6.5 Chapter Summary
6.6 Suggested Further Readings
7. Models for a Combination of Structurally Different and Interchangeable Raters
7.1 Basic Multilevel Models with Correlated First-Order Factors for a Traditional Combination of Different Types of Raters
7.2 Multilevel CTC(M-1) Models for a Combination of Structurally Different and Interchangeable Rate
7.2.1 Application of the multilevel CTC(M-1) model: Loneliness and flourishing
7.2.2 The multilevel CTC(M-1) model as an extension of the trait-reputation-identity model to multiple traits
7.3 Extensions of the models to other measurement designs
7.3.1 Multilevel CTC(M-1) model for multiple sets of interchangeable raters
7.3.2 Multilevel CTC(M-1) model for fully nested structurally different raters
7.3.3 Application of CFA-MTMR models for a special combination of rater groups
7.4 Chapter Summary
7.5 Suggested Further Readings
8.Models for Cross-Classified Multiple Membership Data
8.1 Models for Crossed Designs with Overlapping Sets of Interchangeable Raters
8.2 Models for Crossed Designs with a Combination of Structurally Different and Overlapping Sets of Interchangeable Raters
8.2.1 Application of the cross-classified CTC(M-1) model: Teaching quality and engagement
8.3 Extensions of the models to other measurement designs
8.4 Chapter Summary
8.5 Suggested Further Readings
9. Models for Longitudinal Multirater Data
9.1 Basic Decomposition of Observed Variables
9.2 Basic Multistate-Multirater (MSMR) Model
9.2.1 Testing for measurement invariance across time
9.2.2 Longitudinal Modeling Strategy
9.2.3 Application of the MSMR Model: Loneliness and flourishing
9.2.4 Summary and extensions
9.3 The Correlated States-Correlated (Methods – 1) Model
9.3.1 Application of the CSM (M-1) Model with Indicator-Specific Factors: Loneliness and Flourishing
9.4 The CSC(M-1) Change Model
9.4.1 Application of the CSC(M -1) change model: Loneliness and flourishing
9.5 The Multimethod LST Model
9.5.1 Application of the MM-LST model: Loneliness and flourishing
9.6 Longitudinal Multirater Models for a Combination of Structurally Different and Interchangeable Raters
9.6.1 The latent state combination of methods (LS-COM) model
9.6.2 Application of the LS-COM model
9.6.3 The latent state-trait combination of methods (LST-COM) model
9.6.4 Application of the LST-COM model
9.7 Chapter Summary
9.8 Suggested Further Readings
10. Advanced Topics in Multitrait-Multirater Analysis
10.1 CFA-MTMR Models with Categorical Observed Variables
10.1.1 Practical recommendations
10.1.2 Application of CFA-MTMR models with categorical observed variables
10.2 Explaining Latent Trait and Rater-Specific Factors in CFA-MTMR Models without Bias
10.2.1 Mean structure
10.2.2 Covariance structure
10.3 Predicting External Criterion Variables based on CFA-MTMR Models
10.3.1 Models with only structurally different raters
10.3.2 Models with interchangeable raters
10.3.3 Application
10.4 Chapter Summary
10.5 Suggested Further Readings
11. Recommendations and Outlook
11.1 Choice of a Design
11.1.1 Type of raters
11.1.2 Who rates whom?
11.2. Selection and Training of Raters
11.3 Choice of a Model
11.4 Other Statistical Models for Analyzing Multiple Rater Data
11.4.1 Other models of confirmatory factor analysis
11.4.2 Models of item response theory (IRT)
11.4.3 Latent class analysis (LCA) and latent profile analysis (LPA)
11.4.4 Generalizability theory (GT)
11.5 Sample Size Planning and A Priori Power Analysis
11.5.1 Introduction
11.5.2 Monte Carlo simulation approach
11.5.3 Simulation example
11.6 Chapter Summary
References
Author Index
Subject Index
About the Authors